AI and scholarship:
a manifesto
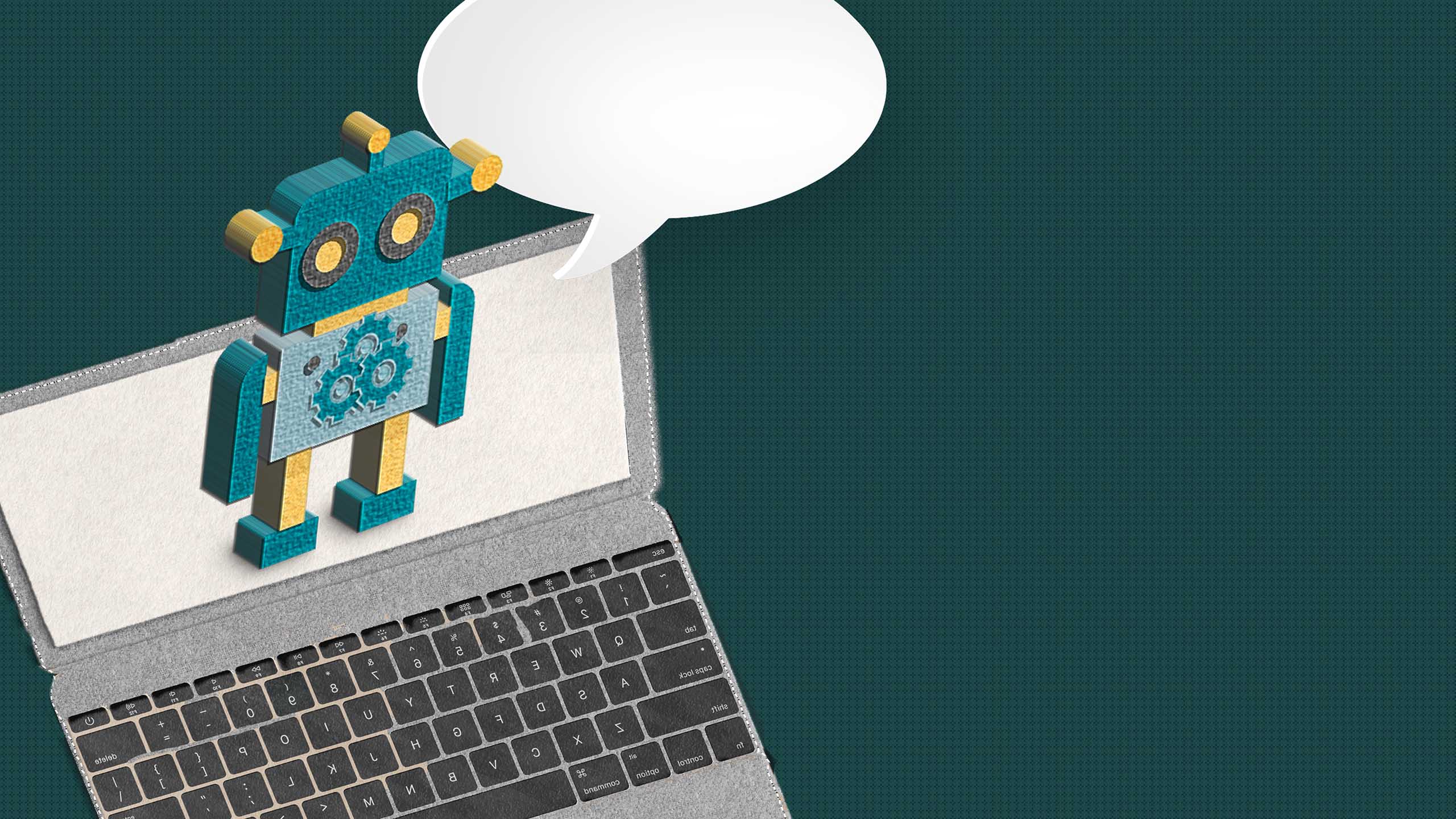
This manifesto and principles cut through the hype around generative AI to provide a framework that supports scholars and students in figuring out if, rather than how, generative AI contributes to their scholarship, writes Dr Ella McPherson and Prof Matei Candea.
This approach reminds us that what is at stake is nothing less than our educational values, they argue.
Introduction
Generative artificial intelligence (AI) has stormed higher education at a time when we are all still recovering from the tragedies and demands of living and working in a pandemic, as well as facing significant workload pressures. It has landed without any significant guidance or resources from a rampant-revenue sector.
For example, ChatGPT’s website provides an eight-(8!)-question ‘Educator FAQ’ which asks for free labour from those who teach to figure out how their technology can ‘help educators and students’: ‘There are many ways to get there, and the education community is where the best answers will come from.’
Still, teaching and teaching support staff have scrambled to find time to carefully think through generative AI’s implications for our teaching and research, as well as how we might address these.
On the teaching side, for example, some colleagues are concerned with generative AI’s potential in enabling plagiarism, while also being excited about generative AI’s prospects for doing lower-level work, like expediting basic computer coding, that makes space for more advanced thinking.
On the research side, we are being pushed various techno-solutions meant to speed up crucial research processes, such as summarising reading, writing literature reviews, conducting thematic analysis, visualising data, writing, editing, referencing and peer reviewing.
Sometimes these options pop up within tools we already use, as when the qualitative analysis software ATLAS.ti launched AI Coding Beta, ‘the beginning of the world’s first AI solution for qualitative insights’, ‘saving you enormous time’.
Saving time – efficiency – is all well and good. But efficiency is not always, or even often, the core norm driving scholarship. The only way to know if and how to adopt generative AI into our teaching, learning and research is through assessing its impact on our main values.
Generative AI and the core values of scholarship
We often hear of academic excellence as the core value of scholarship. The use of generative AI, where it facilitates knowledge generation, can be in line with this core value, but only productively if it doesn’t jeopardise the other values animating our teaching, learning and research.
As described below, these include education, ethics and eureka. We have to broaden the conversation to these other values to fully understand how generative AI might impact scholarship.
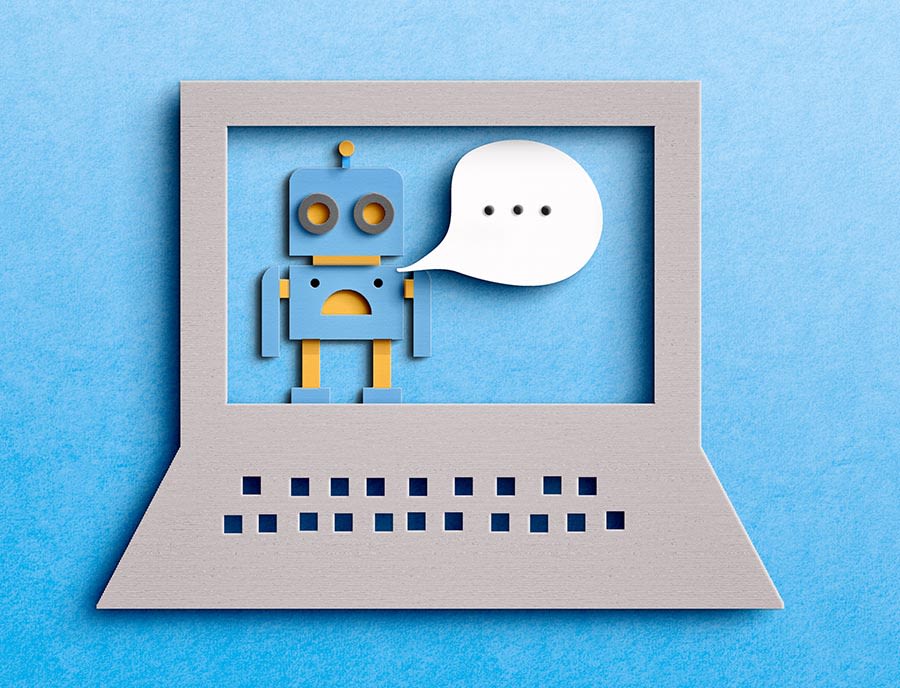
Education
Education is at the heart of scholarship. As students and scholars, understanding the how of scholarship is just as important as the what of scholarship, yet it often gets short shrift. Methodology is emphasised less than substantive topics in course provision, and teaching and learning often focuses more on theories than on how they were made and on how the makings shaped the findings.
This misattention means we have been slower to notice that the adoption of generative AI may take away opportunities to learn and demonstrate the key skills underpinning the construction of scholarship.
Learning-by-doing is a pedagogical approach that applies just as much to the student as the established scholar. It is often slow, discombobulating, full of mistakes and inefficiencies, and yet imperative for creating new scholarship and new generations of scholars.
Though generative AI can support scholarship in some ways, we should be sure that we understand and can undertake the processes generative AI replaces first, such as summarising and synthesising texts, generating bibliographies, analysing data and constructing arguments.
If we allow generative AI, we also have to think about how it impacts the equality of access to education. On the one hand, users who can pay have access to more powerful tools. On the other, educators are investigating the potential for generative AI to support disabled students, though past experience shows us that rushing into AI adoption, like transcription, in the classroom has had significant negative repercussions.
Ethics
The initial enchantment of generative AI also distracted us from the complex ethical considerations around using generative AI in research, including their extractive nature vis-à-vis both knowledge sectors and the environment, as well as the way they trouble important research values like empathy, integrity and validity.
These concerns fit into a broader framework of research ethics as the imperative to maximise benefit and minimise harm.
We are ever more aware that many large language models have been trained, without permission or credit, on the creative and expressive works of many knowledge sectors, from art to literature to journalism to the academy.
Given the well-entrenched cultural norm of citation in our sector – which acknowledges the ideas of others, shows how ideas are connected, and supports readers in understanding the context of our writing – it is uncomfortably close to hypocritical to rely on research and writing tools that do not reference the works on which they are built.
Sustainability is increasingly a core value of our universities and our research. Engaging generative AI means calling on cloud data centres, which means using scarce freshwater and releasing carbon dioxide.
A typical conversation with ChatGPT, with ten to 50 exchanges, requires a half-litre of water to cool the servers, while asking a large generative AI model to create an image for you requires as much energy as charging your smartphone’s battery up all the way. It’s difficult to un-know these environmental consequences, and they should give us pause at using generative AI when we can do the same tasks ourselves.
Research ethics are about conducting research with empathy and pursuing validity, namely producing research that represents the empirical world well, as well as integrity, or intellectual honesty and transparency. Generative AI complicates all of these. Empathy is often created through proximity to our data and closeness to our subjects and stakeholders. Generative AI as the machine-in-the-middle interferes with opportunities to build and express empathy.
The black box nature of generative AI can interfere with the production of validity, in that we cannot know exactly how it gets to the thematic codes it identifies in data, nor to the claims it makes in writing – not to mention that it may be hallucinating both these claims and the citations on which they are based.
The black box also creates a problem for transparency, and thus integrity; at a minimum, maintaining research integrity means honesty about how and when we use generative AI, and scholarly institutions are developing model statements and rubrics for AI acknowledgements.
Furthermore, we have to recognise that generative AI may be trained on elite datasets, and thus exclude minoritised ideas and reproduce hierarchies of knowledge, as well as reproduce biases inherent in this data – which raises questions about the perpetuation of harms arising from its use.
As always with new technologies, ethical frameworks are racing to catch up with research practices on new terrains. In this gap, it is wise to follow the advice of internet researchers: follow your instinct (if it feels wrong, it possibly is) and discuss, deliberate and debate with your research collaborators and colleagues.
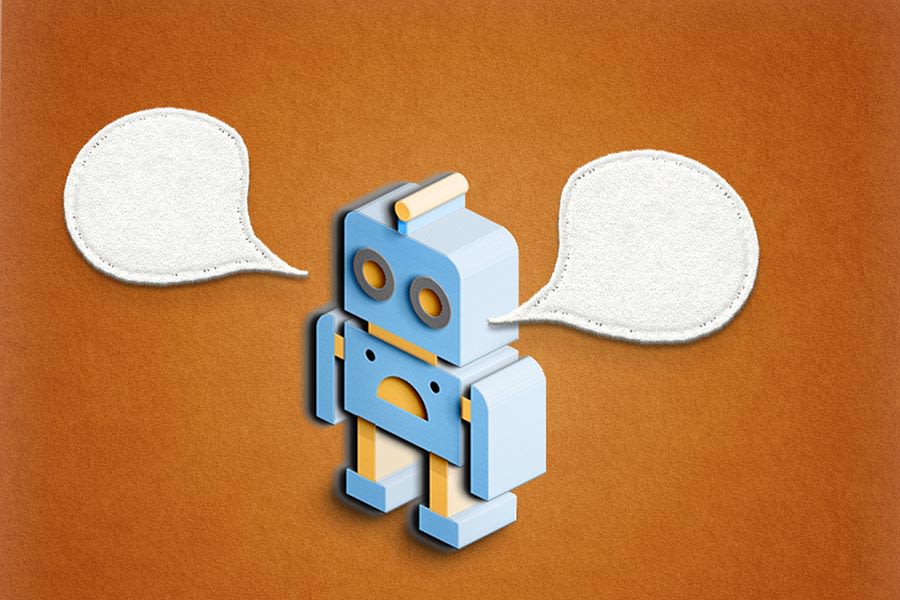
Eurekas
It’s not just our education and our ethics that generative AI challenges, but also our emotions. As academics, we don’t talk enough about how research and writing make us feel, yet those feelings animate much of what we do; they are the reward of the job.
Think of the moment a beautiful mess of qualitative data swirls into theory, or the instant in the lab when it becomes clear the data is confirming the hypothesis, or when a prototype built to solve a problem works, or the idea that surfaces over lunch with a colleague.
These data eurekas are followed by writing eurekas, ones that may have special relevance in the humanities and social sciences (writing is literally part of the methodology for some of our colleagues): the satisfaction of working out an argument through writing it out, the thrill of a sentence that describes the empirical world just so, the nerdy pride of wordplay. Of course, running alongside these great joys are great frustrations, the one dependent on the other.
The point is that these emotions of scholarship are core to scholarship’s humanity and fulfilment. Generative AI, used ethically, can make space for us to pursue them and in so doing, create knowledge. But generative AI can also drain the emotions out of research and writing, parcelling our contributions into the narrower, more automatic work of checking and editing. And this can happen by stealth, with the shiny promise of efficiency eclipsing these fading eureka moments.
Of course, this process of alienation is nothing new when it comes to the introduction of technologies into work, and workers have resisted it throughout time, from English textile workers in the 1800s to Amazon warehouse workers today. As the Luddites were, contemporary movements are often criticised for being resistant to change, but this criticism misses the point. Core to these refusal and resistance movements, as in this case, is noticing what we lose with technology’s gain.
The carrot approach
In the context of a tech-sector fuelled push to adopt new technologies, we argue that the academy should take its time and question not when or how but if we should use generative AI in scholarship.
Rather than being motivated by the stick of academic misconduct, decisions around generative AI and scholarship should be motivated by the carrot of our values. What wonderful and essential values do we protect by doing scholarship the human way? We strengthen our education; protect knowledge sectors, research subjects and principles, as well as the environment; and we make space for eureka moments.
Generative AI has created a knowledge controversy for scholarship. Its sudden appearance has denaturalised the taken-for-granted and has created opportunities for reflection on and renewal of our values – and these are the best measure for our decisions around if and how we should incorporate generative AI into our teaching, learning and research.
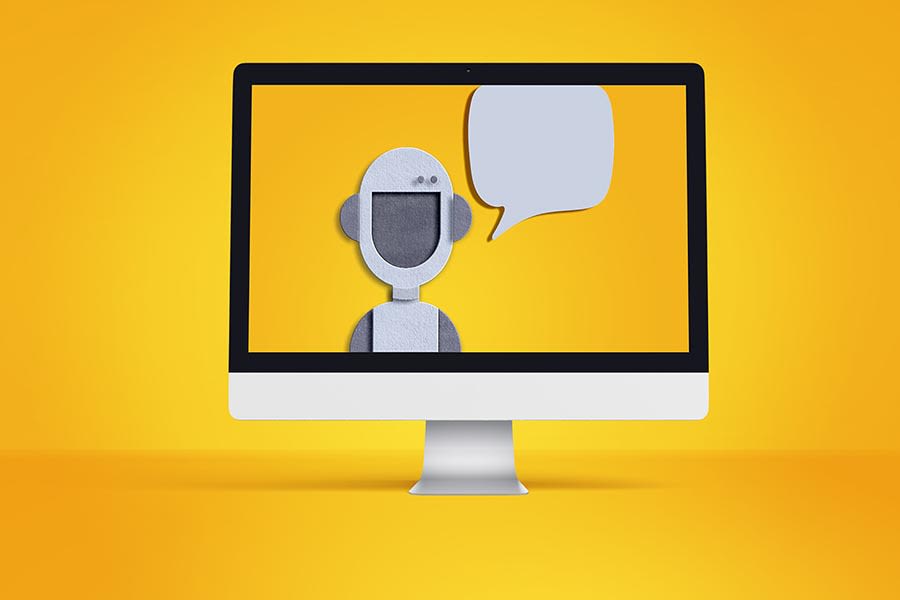
Five Key Principles on AI and Scholarship
Based on the considerations above, we propose these five key principles on AI:
1. Think about it, talk about it.
AI is here to stay. It is increasingly pervasive, embedded in everyday applications and already forms part of staff and student workflows. We need to debate and discuss its use openly with colleagues and students. While we will benefit from technical training and ongoing information on the developing capacities of AI, we as experts in the social sciences and humanities, have a leading role to play in analysing and debating the risks and benefits of AI. We need to make our voice heard.
2. Our values come first.
The values animating our teaching, learning and research must lead and shape the technology we use, not the other way around. We need to pay particular attention to the joys of writing and research, as well as ensure AI enhances these rather than alienates us from them.
3. Stay ethically vigilant.
While the use of AI may be justified or indeed increasingly unavoidable in some cases, we need to remain vigilant as to the way generative AI in particular is extractive vis-à-vis both knowledge sectors and the environment, as well as the way it troubles important research values like empathy, integrity and validity. There is no ethically unproblematic use of AI.
4. Embracing change doesn't mean giving up on the skills we have.
Just because AI seems able to undertake tasks such as summarising and organising information, it doesn't follow that these skills should no longer be taught and assessed. To live in a world full of AI, our students will also need to learn to do without it. This means that, while we are likely to build an engagement with AI in diverse forms of teaching and assessment, zero-AI assessments (such as invigilated exams) will likely remain a core part of our assessment landscape going forward.
5. Be mindful of disciplinary diversity.
AI takes many forms. Some seem relatively benign, speeding up basic tasks, while others take away from students’ ability to learn, or raise deep concerns about authorship and authenticity. Where the line is drawn will depend on different disciplinary traditions, different professional cultures, different modes of teaching and learning. Departments and faculties must have the autonomy to decide which uses of AI are acceptable to them and which are not, in research, teaching and learning.
More information:
Guidelines for AI acknowledgements
Generative AI guidance from the School of Humanities and Social Sciences
This manifesto and principles were prepared for the School of Humanities and Social Sciences (SHSS) by Dr Ella McPherson, Deputy Head and Director of Education (manifesto) and Prof Matei Candea, SHSS Academic Project Director for Technology and Teaching (principles), with support from Megan Capon, SHSS Academic Project Coordinator.
We prepared them at the request of the SHSS Council to support departments and faculties in deciding if and how uses of generative AI are acceptable in their disciplines, research and assessment. These decisions are in line with the University’s designation of the use of AI in assessments as academic misconduct unless expressly allowed and acknowledged.
Published 15 March 2024
Imagery: Carol Yepes / Getty images
The text in this work is licensed under a Creative Commons Attribution 4.0 International License
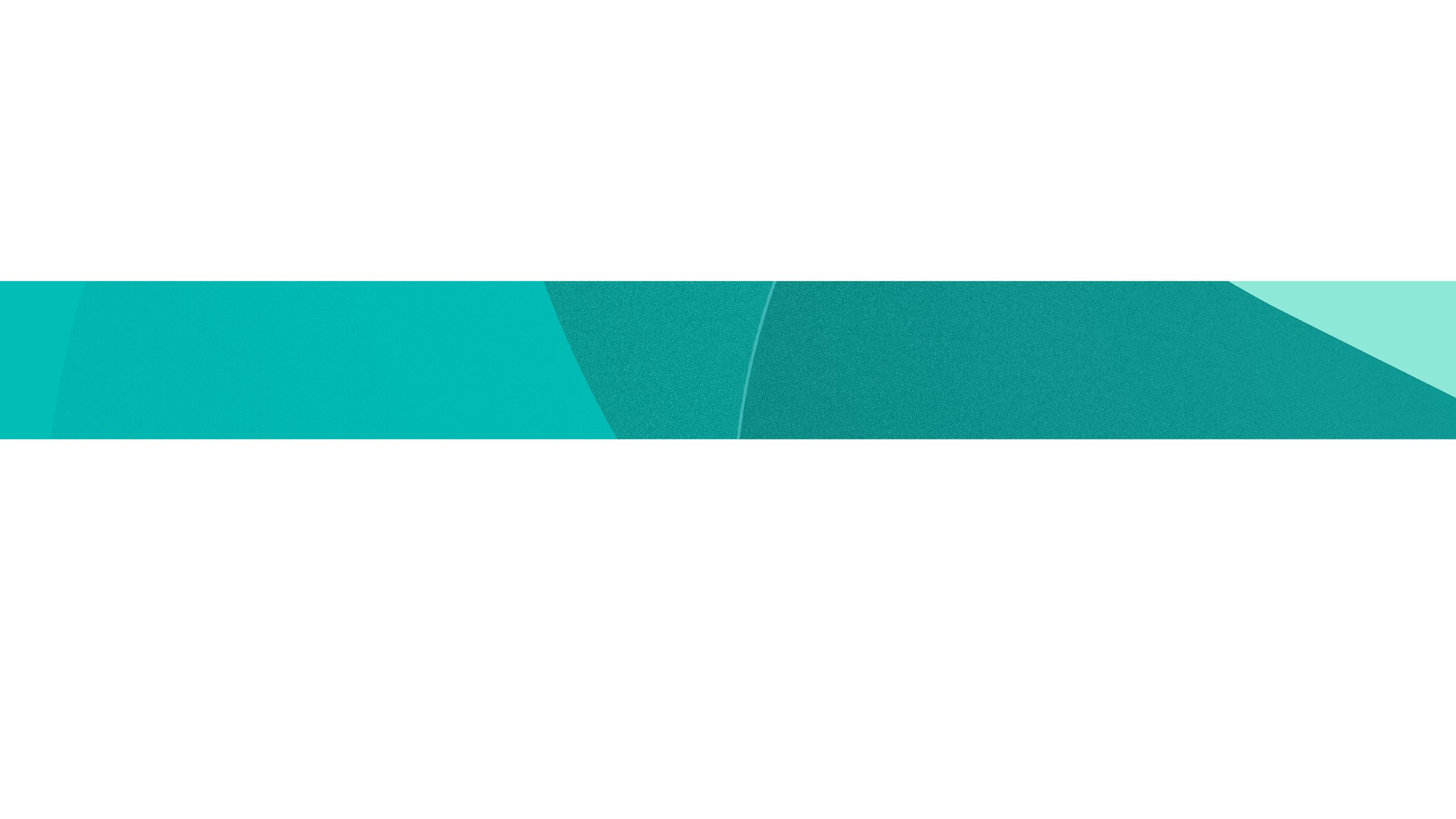